
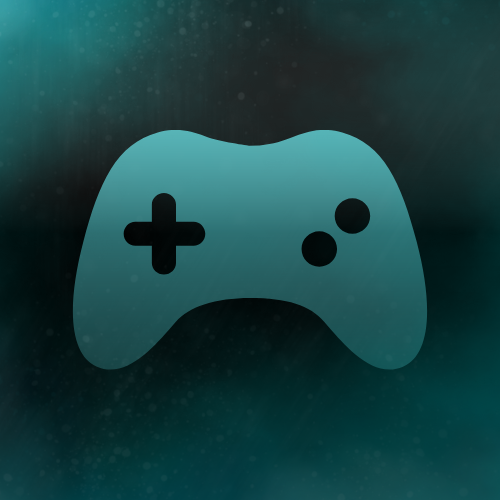
the YouTube experience is far less annoying on average.
Are you sure about that?
I opened YT links without premium on a new browsers and holy moly! I got 1-3 minute unskippable ads every time.
I immediately clicked them off, of course.
the YouTube experience is far less annoying on average.
Are you sure about that?
I opened YT links without premium on a new browsers and holy moly! I got 1-3 minute unskippable ads every time.
I immediately clicked them off, of course.
GN is indeed a rare outlier. They’re like an oldschool tech site that rose at the exact right time to grow up on YouTube.
And our site was like the opposite. Uh… let’s just say many Lemmy users wouldn’t like its editor, but he did not hold back gut punches, and refused to watch his site turn into a clickbait farm.
I briefly wrote articles for an oldschool PC hardware outlet (HardOCP if anyone remembers)… And I’m surprised any such sites are still alive. Mine shut down, and not because they wanted to.
Why?
Who reads written text over their favorite YouTube personality, or the SEO garbage that pops up first on their search, or first party articles/recs on steam, and so on? No one, except me apparently, as their journalistic integrity aside, I’m way too impatient for youtube videos, and am apparently the only person on the planet that believes influencers as far as I can throw them.
And that was before Discord, Tiktok, and ChatGPT really started eating everything. And before a whole generation barely knew what a website is.
They cited Eurogamer as an offender here, and thats an outstanding/upstanding site. I’m surprised they can even afford to pay that much as a business.
And I’m not sure what anyone is supposed to do about it.
Almost all of Qwen 2.5 is Apache 2.0, SOTA for the size, and frankly obsoletes many bigger API models.
To actually answer this, you could look into free APIs of open source models, which have daily limits but are otherwise largely catch-free. You could even mirror endpoints on your VPS if you need to, or host “middleware” like prompt formatters and enhancers.
I say this because, as others said, you cannot actually host AI on a VPS…
It’s still around?
I remember when Star Citizen first popped up, and it makes me feel old.
I feel like you’re attacking the wrong thing.
The subscription hike is something, but U.S./U.K. inflation from 2008 to 2022 is about 40%, and that’s not accounting for any changes in corporate taxes. Its… well, it’s kinda mad that WoW hasn’t increased the subscription price that whole time, if that’s true, but that’s partially because they sell expansions, right? And those probably creep up with inflation.
The problem is the choices they’ve made with that money, aka shoving more aggressive monetization into the game instead of keeping it simple, which was so central to its appeal long ago. Of taking short term profits instead of investing in R&D, new game development, and deeper development for Runescape. This is the real corporate greed. Making money is fine, but just taking it as pure profit at the expense of long-term health is destructive, greedy, unfair to the employees and wrong.
Also, I played Runescape ages ago, and well… I just got tired of the game. I feel like thats why many people left, and I also think it’s kinda mad expecting most players to play the same game forever.
These days, there are amazing “middle sized” models like Qwen 14B, InternLM 20B and Mistral/Codestral 22B that are such a massive step over 7B-9B ones you can kinda run on CPU. And there are even 7Bs that support a really long context now.
IMO its worth reaching for >6GB of VRAM if LLM running is a consideration at all.
I am not a fan of CPU offloading because I like long context, 32K+. And that absolutely chugs if you even offload a layer or two.
For local LLM hosting, basically you want exllama, llama.cpp (and derivatives) and vllm, and rocm support for all of them is just fine. It’s absolutely worth having a 24GB AMD card over a 16GB Nvidia one, if that’s the choice.
The big sticking point I’m not sure about is flash attention for exllama/vllm, but I believe the triton branch of flash attention works fine with AMD GPUs now.
Basically the only thing that matters for LLM hosting is VRAM capacity. Hence AMD GPUs can be OK for LLM running, especially if a used 3090/P40 isn’t an option for you. It works fine, and the 7900/6700 are like the only sanely priced 24GB/16GB cards out there.
I have a 3090, and it’s still a giant pain with wayland, so much that I use my AMD IGP for display output and Nvidia still somehow breaks things. Hence I just do all my gaming in Windows TBH.
CPU doesn’t matter for llm running, cheap out with a 12600K, 5600, 5700x3d or whatever. And the single-ccd x3d chips are still king for gaming AFAIK.
To go into more detail:
Exllama is faster than llama.cpp with all other things being equal.
exllama’s quantized KV cache implementation is also far superior, and nearly lossless at Q4 while llama.cpp is nearly unusable at Q4 (and needs to be turned up to Q5_1/Q4_0 or Q8_0/Q4_1 for good quality)
With ollama specifically, you get locked out of a lot of knobs like this enhanced llama.cpp KV cache quantization, more advanced quantization (like iMatrix IQ quantizations or the ARM/AVX optimized Q4_0_4_4/Q4_0_8_8 quantizations), advanced sampling like DRY, batched inference and such.
It’s not evidence or options… it’s missing features, thats my big issue with ollama. I simply get far worse, and far slower, LLM responses out of ollama than tabbyAPI/EXUI on the same hardware, and there’s no way around it.
Also, I’ve been frustrated with implementation bugs in llama.cpp specifically, like how llama 3.1 (for instance) was bugged past 8K at launch because it doesn’t properly support its rope scaling. Ollama inherits all these quirks.
I don’t want to go into the issues I have with the ollama devs behavior though, as that’s way more subjective.
It’s less optimal.
On a 3090, I simply can’t run Command-R or Qwen 2.5 34B well at 64K-80K context with ollama. Its slow even at lower context, the lack of DRY sampling and some other things majorly hit quality.
Ollama is meant to be turnkey, and thats fine, but LLMs are extremely resource intense. Sometimes the manual setup/configuration is worth it to squeeze out every ounce of extra performance and quantization quality.
Even on CPU-only setups, you are missing out on (for instance) the CPU-optimized quantizations llama.cpp offers now, or the more advanced sampling kobold.cpp offers, or more fine grained tuning of flash attention configs, or batched inference, just to start.
And as I hinted at, I don’t like some other aspects of ollama, like how they “leech” off llama.cpp and kinda hide the association without contributing upstream, some hype and controversies in the past, and hints that they may be cooking up something commercial.
Nah, I should have mentioned it but exui is it’s own “server” like TabbyAPI.
Just run exui on the host that would normally serve tabby, and access the web ui through a browser.
If you need an API server, TabbyAPI fills that role.
Shrug did you grab an older Qwen GGUF? The series goes pretty far back, and its possible you grabbed one that doesn’t support GQA or something like that.
Doesn’t really matter though, as long as it works!
Your post is suggesting that the same models with the same parameters generate different result when run on different backends
Yes… sort of. Different backends support different quantization schemes, for both the weights and the KV cache (the context). There are all sorts of tradeoffs.
There are even more exotic weight quantization schemes (ALQM, VPTQ) that are much more VRAM efficient than llama.cpp or exllama, but I skipped mentioning them (unless somedone asked) because they’re so clunky to setup.
Different backends also support different samplers. exllama and kobold.cpp tend to be at the cutting edge of this, with things like DRY for better long-form generation or grammar.
So there are multiple ways to split models across GPUs, (layer splitting, which uses one GPU then another, expert parallelism, which puts different experts on different GPUs), but the way you’re interested in is “tensor parallelism”
This requires a lot of communication between the GPUs, and NVLink speeds that up dramatically.
It comes down to this: If you’re more interested in raw generation speed, especially with parallel calls of smaller models, and/or you don’t care about long context (with 4K being plenty), use Aphrodite. It will ultimately be faster.
But if you simply want to stuff the best/highest quality model you can at VRAM, especially at longer context (>4K), use TabbyAPI. Its tensor parallelism only works over PCIe, so it will be a bit slower, but it will still stream text much faster than you can read. It can simply hold bigger, better models at higher quality in the same 48GB VRAM pool.
It’s probably much smaller than whatever other GGUF you got, aka more tightly quantized.
Look at the filesize, thats basically how much RAM it takes.
Joke’s on the websites, as I run Cromite, so no pop ups or anything.
…But also, most of the written web is trash now.
:(